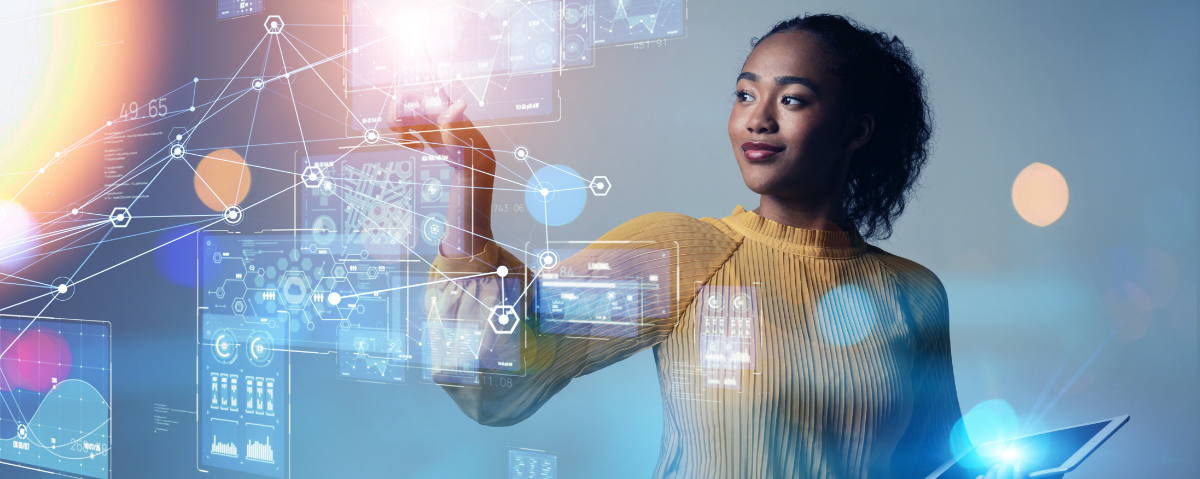
Making important decisions is difficult. Before the modern advances in data collection and analysis, people made decisions based on qualitative factors, ad hoc assessments and, more often than not, based on gut feel.
The history of the world is plagued by misfortunes derived from incomplete insights leading to poor decisions. Even though some of those ended up with positive outcomes.
One example: In 1492, Christopher Columbus sailed west in the pursuit of finding an alternative and shorter route to the Indies. We all know how the story ends up but just for some perspective, he didn’t take that decision lightly; he researched and embraced the high technology of his time, learning math, celestial navigation or shipbuilding, and (together with his brother, apparently), he founded a map-making business in Lisbon which put him in touch with ideas and trends from all over Europe.
Christopher Columbus, Big Data User
It was the revolutionary equivalent of today’s Big Data and AI technologies, which at the time were also generating huge discoveries and massive fortunes. It’s proven that the discovery of America was a lucky event and less a natural outcome proportionate to all Columbus’ knowledge, because his expedition was almost trumped by terrible miscalculations:
- He used bad data: In 200 BCE, Eratosthenes very accurately calculated the circumference of the earth. One degree of latitude was equal to 59.5 nautical miles, but later estimates by medieval Alfraganus suggested 56.67 nautical miles, the number Columbus assumed.
- He used bad calculations: He compounded the error by 25% by assuming Alfraganus was using Roman miles while he was actually using the Arabic mile. We shouldn’t laugh at a 500-year-old mistake; a similar units-of-measurement error proved fatal for the Mars Climate Orbiter in 1999.
- He made bad assumptions: Columbus also assumed that Asia was stretched out eastward and almost touching the Azores. In short, he wanted the data to fit his narrative by adding his own reasoning to what was known via Ptolemy and Marco Polo, which led to an additional 60% of margin error.
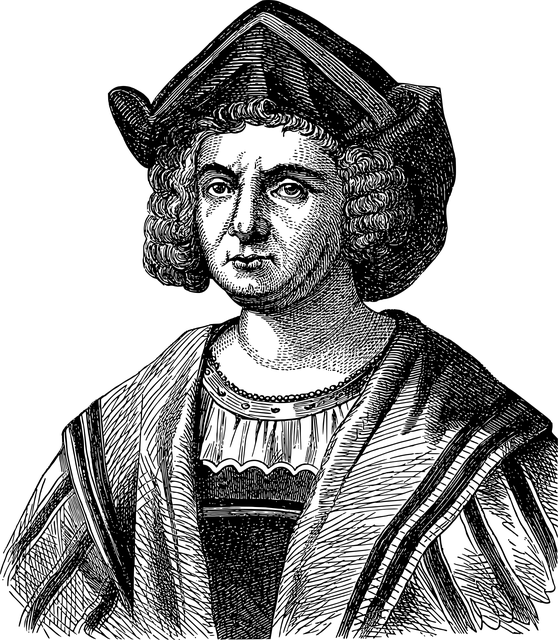
Compounding a Cognitive Bias
Overall, the ultimate issue was that Columbus did not show an ability or willingness to assess the true value of prior decisions, history, and experience, allowing these to get in the way. Personal judgment, experience, and gut feelings compounded a strong cognitive bias based on his rich maritime knowledge.
We can’t blame Columbus for making those decisions given the limited available amount of true knowledge. He ended up getting lucky, and the unintended consequences of poor decision-making clearly yielded an incredible ROI for the Spanish Crown. But this example showcases how flawed cognitive bias can be, and the impact that it had on what was actually a very risky decision.
Obviously, we live now in different times. But the situation is very relatable to all of us when having to make decisions; we are often in the same situation of having incomplete or bad data, and we fit our decision-making process into the data rather than do the opposite. Data is one of the most valuable resources any business could have, and although “errors using inadequate data are much less than using no data at all,” according to Charles Babbage, we always need to approach these decisions with caution.
Data Quality is the Foundation of Effective Business Intelligence
Making a data-driven decision is a process where you collect data and extract patterns, facts, or insights from it to make inferences that guide your final decision. But the data could be inaccurate, historical results may not be a good indicator of the future, and models using simplifying assumptions could be unreliable.
To be more specific, bad data is any piece of information that is erroneous, misleading, or confusing in its format. Examples of bad data are incomplete data, which won’t provide the complete picture; inaccurate data that misrepresents a situation, thereby not allowing you to target or define strategies accordingly; obsolete data that occurs because, in a modern and digital ecosystem, changes happen so quickly that even information that looks representative it might be irrelevant; and duplicate data that skews metrics, slows down processing and storage, and more.
In the era of the digitally-engaged customer, the quality of the customer experience is what defines a business’s success. Since a substantial portion of customer engagement happens online, it’s imperative that your company has a 360-degree-view of your customer to gain a competitive edge.
With the advancement of the digital world and Internet of Things, massive amounts of information are being captured on every customer interaction. So the need for depurating, arranging, and distilling insights is more important than ever.
“Data, data everywhere, but not a thought to think.” – John Allen Paulos
Most people would say that they believe that a lot of data is good, while the reality is that this is far from the truth unless it has been properly sanitized. Many organizations acknowledge this success factor but rarely act upon it. So regardless of how “big” data is, quality is always more important than quantity.
Qualities of Good Data
- Completeness: There are no gaps in the data; everything that was supposed to be collected was done so successfully.
- Relevancy: The information is actually useful for your initiatives, objectives, and decisions. Even if the data is high quality, it’s useless if it’s irrelevant to your goals.
- Accuracy: The data you have describes real business conditions that help you draw the correct conclusions.
- Consistency: Each data item is uniform in content and format with their counterparts across multiple datasets and databases.
- Timeliness: Data reflects current reality and is captured immediately after the event.
Developing the Right Methods for Analyzing Data
We see very often that some companies get too paralyzed in their efforts to undertake a data readiness assessment for digital transformation. The reality is that typically, information already available tends to be good enough, but the main challenge is to dissect it, integrate it with other environments, and deliver it at the right place in the right format at the right time.
As business leaders, we need to understand the shortcomings of quantitative analysis and incorporate qualitative factors into our decision-making process.
We also need to ensure we have an effective, efficient data integration framework across commercial capabilities and wider business functions. This is a critical prerequisite for success that prevents us from making wrong decisions that are not only driven by bad assumptions, but also from not being able to measure and assess our course in due time so we can adjust it as we go.
“Our doubts are traitors, and make us lose the good we oft might win, by fearing to attempt.” —William Shakespeare
A good way of preventing this is to use the data signals from your commercial processes and the market to build a more complete picture of your customers and then ensure you manage your pricing and commercial processes accordingly such as being able to tie Customer lifecycle, product lifecycle, to commercial decisions while reacting to market dynamics and moods.
Don’t be frozen in analysis. A key objective of data science is correcting cognitive bias. As humans, we have an alarming lack of statistical intuition and are prone to confuse correlation with causation.
AI is a Better Data Navigator
Nowadays, quantitative techniques and AI allow you to interpret large datasets and implement changes faster. Analyzing data very often increases efficiency, but also helps identify new business opportunities that may have been otherwise overlooked, such as untapped customer segments. In doing so, the potential for growth and profitability becomes endless and more intelligence-based
Knowledge about your customers’ spending patterns, price sensitivity, and behaviors is essential for establishing and continuously developing your business strategy. If your data is inaccurate, dated, or incomplete, your tactics won’t be as effective and you might miss out on potential prospects.
Best Practices for Good Data-Driven Decision-Making
The conclusions you draw from a data-driven decision-making process can assist you in making more informed decisions and driving more successful strategies forward.
Every wrong business decision is a good opportunity missed. Knowledge about your customers’ buying patterns, price sensitivity, and behavior is essential for establishing and continuously developing your commercial tactics. If your data is inaccurate, obsolete, or incomplete, your strategies won’t be as effective and you might leave profit on the table or even miss out on potential prospects.
Even worse, you won’t even realize what the problem is because your data won’t tell you that it’s wrong. You won’t be able to solve the problem or optimize your strategy. You might also lose your advantages over competitors because you were unable to anticipate the needs of your prospects.
The short-term and long-term decisions you plan to make is tied to the quality of business data you have. Although it can be time-consuming to maintain data health and make decisions like this, it will benefit your business in the long run.
- Identify your business goals and understand the user. Every user should know the business well and understand what the organization wants. That will help later on to connect insights into decisions aligned with business objectives.
- Accurate data is the foundation of meaningful analysis. By definition, statistical work that analyzes flawed data will also be flawed and much more likely to lead to a poor decision than an analysis based on accurate data. Find reliable data sources and establish how to clean, organize, and maintain the data so it can be easily accessible and presentable. This tends to be a very time-consuming task, but your investments in this area will pay off handsomely later.
- Don’t stop on data warehousing and DB administration. Refine your data collection process. Quality data is a result of an ongoing effort; it won’t work with just a one-time cleanse. All the information you have should be properly collected, processed, and managed, especially as your business evolves
- Historical data may not be a good indicator of the future. Perform statistical analysis, define control groups and sampling techniques to validate and confirm your assumptions. The ability to effectively determine the key factors explaining changes is critical but remember that correlation and causation are two different things
- Metrics that simplify complex business processes should be developed with caution. Everyone likes a simple metric to guide decision-making. The issue is that this approach has the potential to oversimplify matters into a single-minded approach and take for granted its assumptions are true as well, failing to consider other qualitative factors in the decision-making.
- Expensive data infrastructure investment or “very advanced AI algos” could lead to too much reliance on data. These investments can then influence leaders to over-rely on this information as part of their decision-making processes, even when other approaches should be used.
- Challenge your assumptions and the cognitive bias at every stage. The last step in the decision-making process is to draw your conclusions from any information or insights you learned from the model. A good place to start is to ask yourself questions you think you already know the answer to. Many companies make assumptions such as “X type of customers are our best customers” or “customers are very price-sensitive on X product”. By challenging these assumptions, you can either confirm them with data or correct them with your new learning.
- Objectivity and honesty are essential to any analysis. We all inherently want to hear a favorable story and/or a simple and easy explanation we can understand .While effective advisers will appreciate this desire, they will also look to develop an accurate story rather than just painting a favorable story that may appeals more to a leader.
- Choose visualization tools that tight insights into decisions. Reduce the path to decision-making to an informed path of least resistance.